In an era where environmental, social, and governance (ESG) considerations are not just ethical imperatives but strategic business advantages, data-driven insights become the backbone of sustainability efforts. By harnessing the capabilities of Retrieval Augmented Generation (RAG) systems, at the forefront of generative AI technology, businesses have the opportunity to transform their approach to ESG initiatives and unlock substantial value.
Within the Intelligent Document Processing field, generative AI technologies like RAG systems are transforming how organizations manage, search, and analyze vast amounts of textual data. The essence of RAG is its ability to retrieve information from a database or a set of documents to generate new, coherent, and contextually relevant text based on a given query. This retrieval-augmented approach combines the best of information retrieval and text generation, enabling the system to provide answers that are not just accurate but also rich in context.
The application of RAG systems in Intelligent Document Processing, particularly for parsing complex documents like ESG reports, is a game-changer. ESG reports are dense, filled with crucial data points that often get buried under volumes of information. RAG systems, with their dual prowess in retrieving and generating information, can sift through these reports, extract pertinent data, and answer queries with unparalleled precision and depth.
To fully appreciate what this innovative approach brings to ESG report analysis through RAG systems, it is essential to delve deeper into the basic building blocks of a RAG system. A RAG system represents the fusion of two primary components: a retrieval component and a generative component. Each plays a critical role in how the system functions, from processing input queries to generating insightful, contextually relevant responses.
The retrieval component is the “search engine” of a RAG system. It scours a large database or document collection to find pieces of information that are relevant to the input query. This component relies on sophisticated algorithms and indexing methods to efficiently search through vast amounts of data. The performance of the retrieval component is paramount, as it directly influences the quality of the information passed to the generative component for the next phase of processing. Techniques such as vector similarity searches, where queries and documents are converted into vectors (points in a multidimensional space) to measure their similarity, are commonly employed.
Integral to the retrieval component is the context database or knowledge base it searches through. This database is a structured collection of documents or data that the RAG system can draw upon to find relevant information. For ESG reporting, this might include a comprehensive repository of ESG reports, regulatory documents, industry benchmarks, and related literature. The organization and quality of this database significantly impact the system’s ability to retrieve accurate and relevant information.
Once relevant information is retrieved, the generative component comes into play. This part of the RAG system uses the retrieved information to construct or generate a coherent, detailed answer to the input query. The generative component leverages advanced natural language processing (NLP) models, such as those based on transformer architectures like GPT (Generative Pre-trained Transformer), to produce responses that are not just accurate but also contextually rich and nuanced. It synthesizes the retrieved data in a way that mirrors human-like understanding and articulation.
A vital building block of a RAG system, especially in specialized applications like ESG reporting, is the fine-tuning mechanism. This allows the system to be customized or tailored to understand and generate responses in a domain-specific context better. Through fine-tuning, the system learns the intricacies of ESG language, concepts, and reporting standards, enabling it to provide more precise and relevant insights.
How does this translate in practice? Imagine querying the system about a company’s carbon footprint or its diversity initiatives. The RAG system can quickly locate relevant information from the ESG reports, generate a summary of findings, and even benchmark these against industry standards or regulatory requirements. This instantaneous access to synthesized and actionable insights drastically reduces research time and empowers decision-makers to act swiftly and confidently.
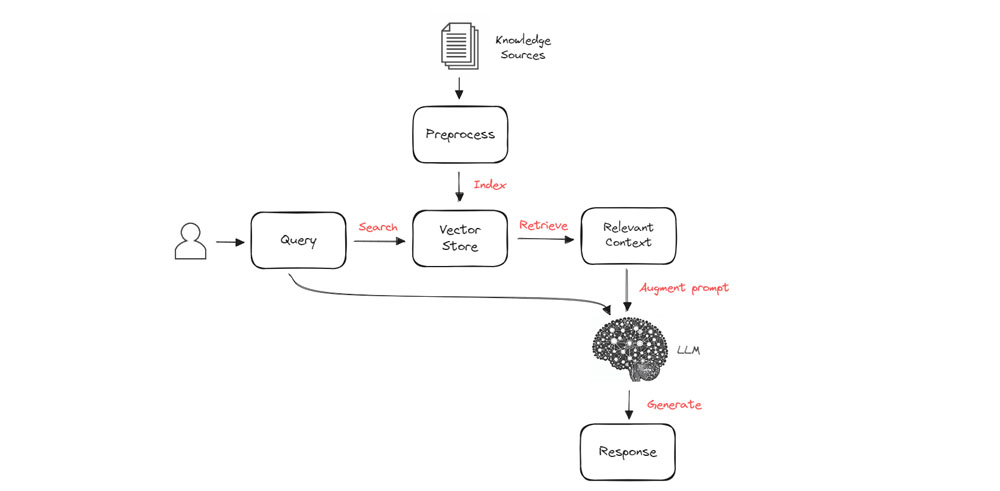
The advantages of utilizing a RAG system for ESG reporting are multifold. Firstly, it improves the efficiency and accuracy of data retrieval, making the process of compiling ESG disclosures noticeably smoother. Secondly, it enhances transparency and accountability, as stakeholders can easily seek and find specific information. Lastly, it fosters informed decision-making, ensuring businesses are not just compliant with ESG regulations but are genuinely contributing to sustainable and ethical practices.
The integration of RAG systems into the analysis of ESG reports marks the beginning of a new era in sustainable business practices. By making ESG data more accessible and actionable, AI empowers organizations to prioritize sustainability in a way that’s informed, effective, and aligned with their strategic goals. This technology stands at the intersection of AI innovation and sustainable development, charting a course towards a more accountable and transparent business world.
Embark on this revolutionary journey with us, and together, let’s redefine the future of ESG reporting.
UHURA IS AN AI PLATFORM THAT READS AND UNDERSTANDS COMPLEX DOCUMENTS JUST AS HUMANS DO. WE HELP BUSINESSES SPEED UP THE REVIEW AND DECISION-MAKING PROCESSES BY USING AI TO UNCOVER VALUABLE INSIGHTS FROM DOCUMENTS, REPORTS, CONTRACTS AND AGREEMENTS. WE USE CUTTING-EDGE AI, INCLUDING IMAGE PROCESSING, NATURAL LANGUAGE PROCESSING AND MACHINE LEARNING TECHNOLOGY, TO BRING UNPRECEDENTED ACCURACY AND SHORTEN DOCUMENT PROCESSING TIME FROM HOURS TO SECONDS.