AI is progressively transforming industries by automating business operations, but along with its advancements, concerns about transparency and accountability have emerged. The concept of AI being shrouded in a black box, with obscure decision-making processes and unexplainable outcomes, has raised scepticism among users and regulatory bodies. But is there a way where AI and transparency go hand in hand?
While concerns about AI transparency persist, end-to-end solutions and transparency-focused approaches are transforming the landscape. The marriage of technology and accountability brings us closer to the realisation that AI systems can be understandable, accessible, and trustworthy.
Transparency as the Key Driver of End-to-End Solutions
End-to-end AI solutions encompass the entire AI development pipeline, from data collection and preprocessing to model training, deployment, and post-deployment monitoring. Unlike fragmented approaches, end-to-end solutions provide a holistic view of the AI system, enabling transparency and traceability throughout the process. By integrating different stages into a unified framework, these solutions offer greater visibility into the inner workings of AI models, allowing users to understand the rationale behind decisions and ensure accountability.
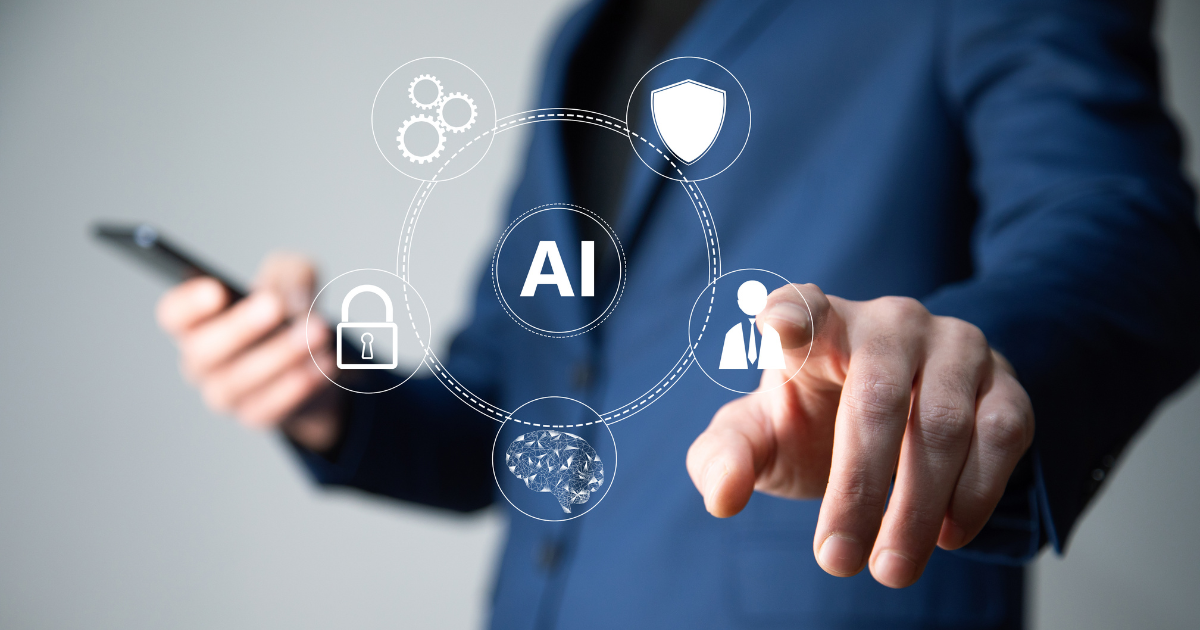
Users gain insights into the data used for training, the features influencing predictions, and the model’s decision-making process. This transparency not only helps users comprehend how AI is impacting their business, but it also aids in identifying biases, errors, or potential risks associated with the system.
Why Low-Code and No-Code Capabilities are so popular?
In the past, deploying AI solutions into businesses typically required extensive technical expertise, specifically in programming and data science. This created a barrier for individuals with diverse backgrounds who lacked the necessary skills to actively participate in the AI adoption process.
However, the emergence of end-to-end solutions has addressed this challenge. These solutions leverage low-code and no-code platforms, which offer intuitive interfaces for creating, modifying, and deploying AI models.
Low-code platforms provide a visual environment that allows users to employ applications using a visual interface with minimal coding. No-code platforms take this a step further by enabling users to harness applications without writing any code at all. These platforms abstract away much of the underlying complexity, making it easier for individuals without extensive programming knowledge to engage with AI.
AI-based low-code and no-code solutions are gaining popularity due to their ability to combine the power of artificial intelligence with the simplicity of visual development. These platforms allow non-technical users to create applications and automate processes without writing code. However, as AI becomes an integral part of these solutions, it is crucial to prioritise transparency and accountability to ensure responsible and ethical use.
Turning point of building trust in AI
One of the key principles in AI-based low-code and no-code solutions is explainable AI (XAI). This means that the AI models should be designed in a way that their decision-making process can be understood and interpreted by users. Transparency in AI algorithms enables users to gain insights into how the AI is making predictions or recommendations. By understanding the factors influencing AI outputs, users can trust the system more and identify potential biases or errors.
In addition to being transparent about AI algorithms and data usage, platforms should provide users with information about the performance metrics of AI models. Metrics such as accuracy, precision, recall, and F1-score allow users to assess the reliability of AI-generated outputs. Knowing the performance metrics helps users understand the AI’s strengths and limitations in various scenarios.
Furthermore, regular audits of AI models are necessary to ensure ongoing compliance with transparency and accountability standards. These audits help identify any issues or biases that may have emerged over time. If problems are detected, they should be promptly addressed, and users affected should be informed about the corrective actions taken.
User understanding plays a crucial role in promoting transparent and accountable AI usage, as well. Users should be informed about the presence of AI and its role in the platform. Transparent communication helps users to comprehend the benefits and limitations of AI and encourages responsible usage.
To foster responsible AI development, transparent platforms should also openly acknowledge the limitations of AI-based automation. There should be awareness about the scenarios where human intervention is necessary and when AI might not be the best solution. Being aware of these limitations ensures users do not rely blindly on AI and make informed decisions.
Finally, establishing user feedback and grievance mechanisms is essential for AI-based low-code and no-code solutions. These mechanisms allow users to provide feedback, report concerns, and raise grievances related to AI-based functionalities. Platform providers should be responsive to user input and take appropriate actions to address concerns.
Making AI a valuable asset
Transparent and accountable practices are paramount in AI-based low-code and no-code solutions to ensure the responsible and ethical use of artificial intelligence. By focusing on explainable AI, performance metrics, audits, user understanding, acknowledgement of limitations, and feedback mechanisms, these platforms can foster user trust and contribute to the development of AI systems that benefit society while mitigating potential risks.